Master projects
Investigating Generative Approaches in the Context of Brain Tumor Magnetic Resonance Imaging (MRI) Scans
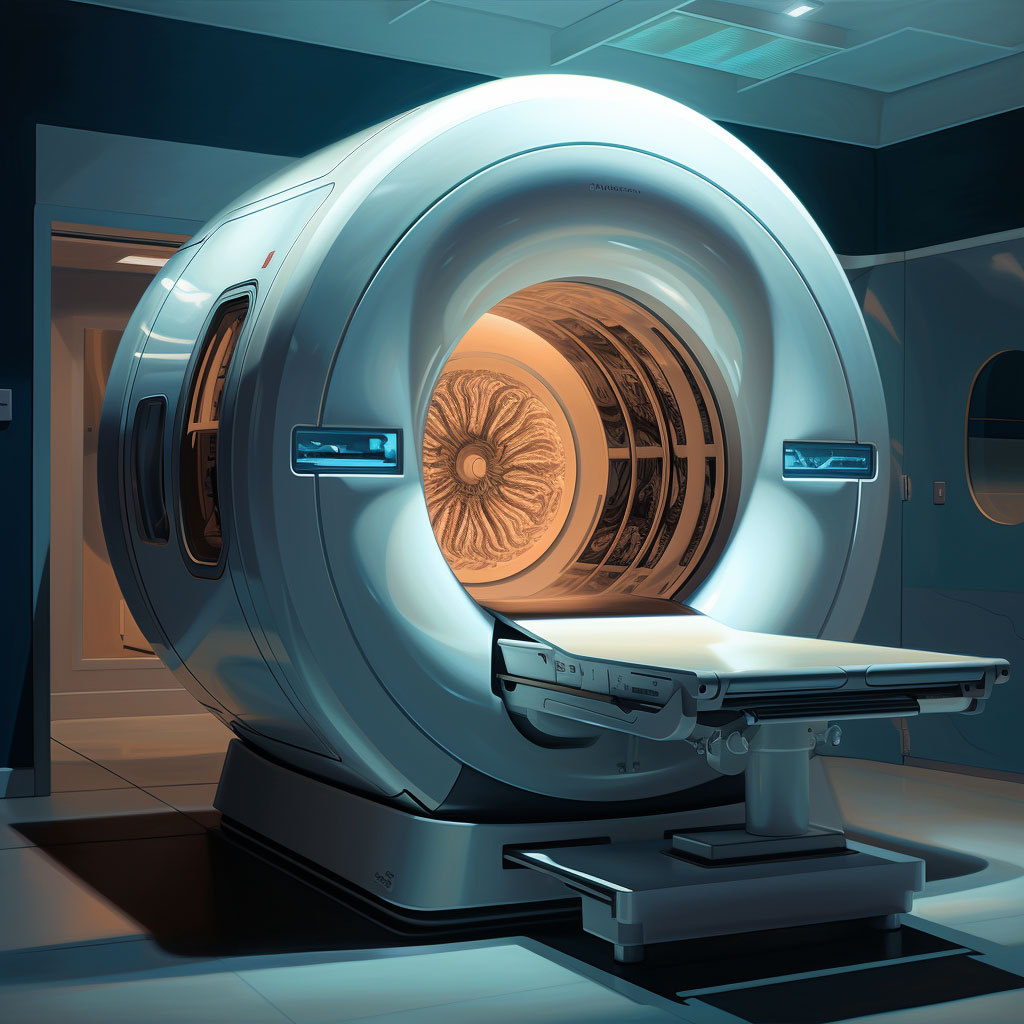
Student
Daria Laslo
external page LinkedIn Daria Laslo
Supervisor
Sarah Brüningk
Summary
Substantial progress has been observed in the performance of generative models, specifically denoising diffusion models, with potential implications for their application in medical data. The Thesis of Daria focuses on implementing a diffusion-based approach to various image-to-image translation tasks in neuro-oncology. One aspect involves using a diffusion model guided by a classifier to generate healthy brain slices starting from diseased ones. Simultaneously, a similar model guided by a regressor can be applied to modify the size of the tumor in the generated image, simulating disease progression. The primary objective of this project is to produce an anatomically relevant model which can reliably generate high-quality and realistic synthetic brain tumor MRI scans showing tumor enlargement.
Prediction of Paediatric Anticancer Drug Sensitivity from Multi-omics Inputs
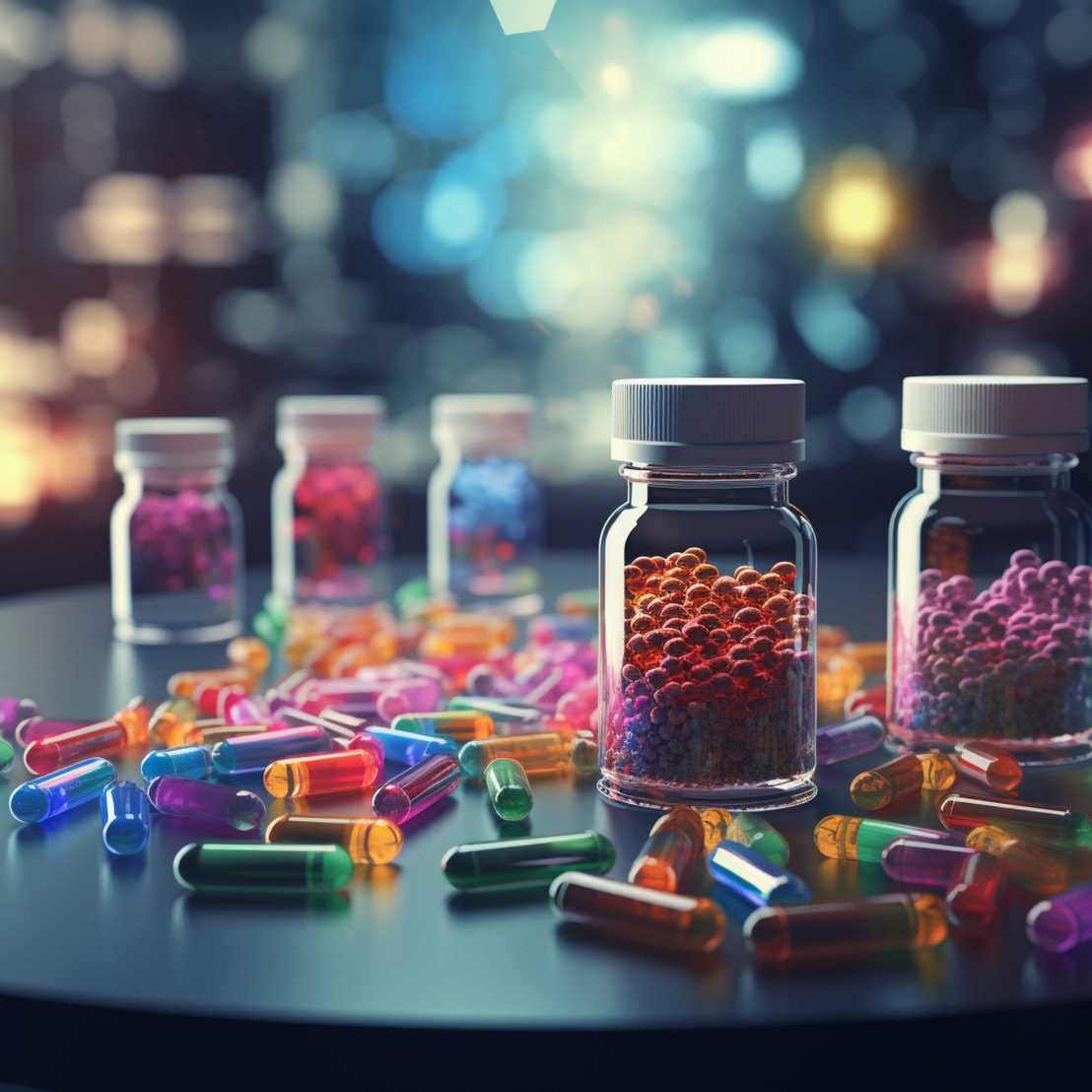
Student
Hui Jeong
external page LinkedIn Hui Jeong
Supervisor
Sarah Brüningk, Catherine Jutzeler
Summary
In her masters thesis, Hui investigates state of the art models for the prediction of drug sensitivity in adult and pediatric cancer cell lines. While adult cancer cell lines have been extensively studied and modeled, paediatric cancer focused models are rare. Recently, a new paediatric cancer cell line multi-omics dataset has been released. This dataset includes DNA sequencing, RNA sequencing, DNA methylation, and pharmacological and genetic CRISPR-Cas9 loss of function screen data. The aim of this project is to characterize and predict paediatric cancer drug sensitivity from these multi-omics inputs and to contrast these against adult tumor drug response.
Temporal Patterns in Spinal Cord Injury Recovery
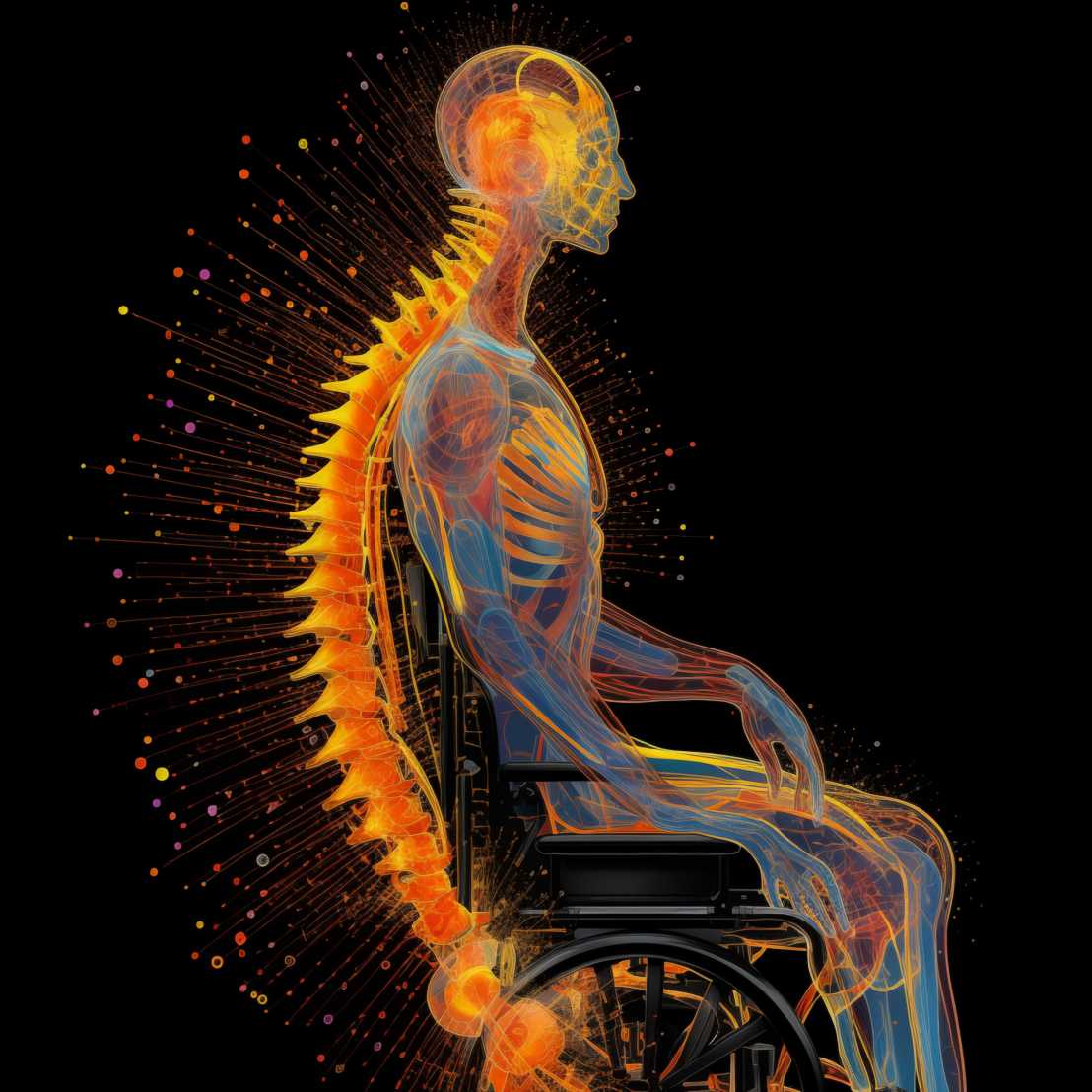
Student
Maya Louage
external page LinkedIn Maya Louage
Supervisor
Lucie Bourguignon
Summary
In her masters thesis, Maya investigates the relation between time of injury and spinal cord injury (SCI) recovery by employing novel statistical tools in hypothesis testing within the SCI field. In her masters thesis, Maya investigates the relation between time of injury and spinal cord injury (SCI) recovery by applying previously unused statistical tools in the SCI hypothesis testing field. The motivation behind investigating the time of injury hypotheses is multifaceted. Firstly, trauma injuries often exhibit "off-hour effects," linked to treatment complications. Secondly, infections are known to hinder SCI recovery, prompting an examination of potential seasonal effects due to infections' seasonal patterns. Lastly, Maya analyzes the recovery of injuries during the COVID period, considering the surge in infections and potential disruptions in rehabilitation access during this time.
Exploring novel metrics for assessing and predicting recovery after spinal cord injury
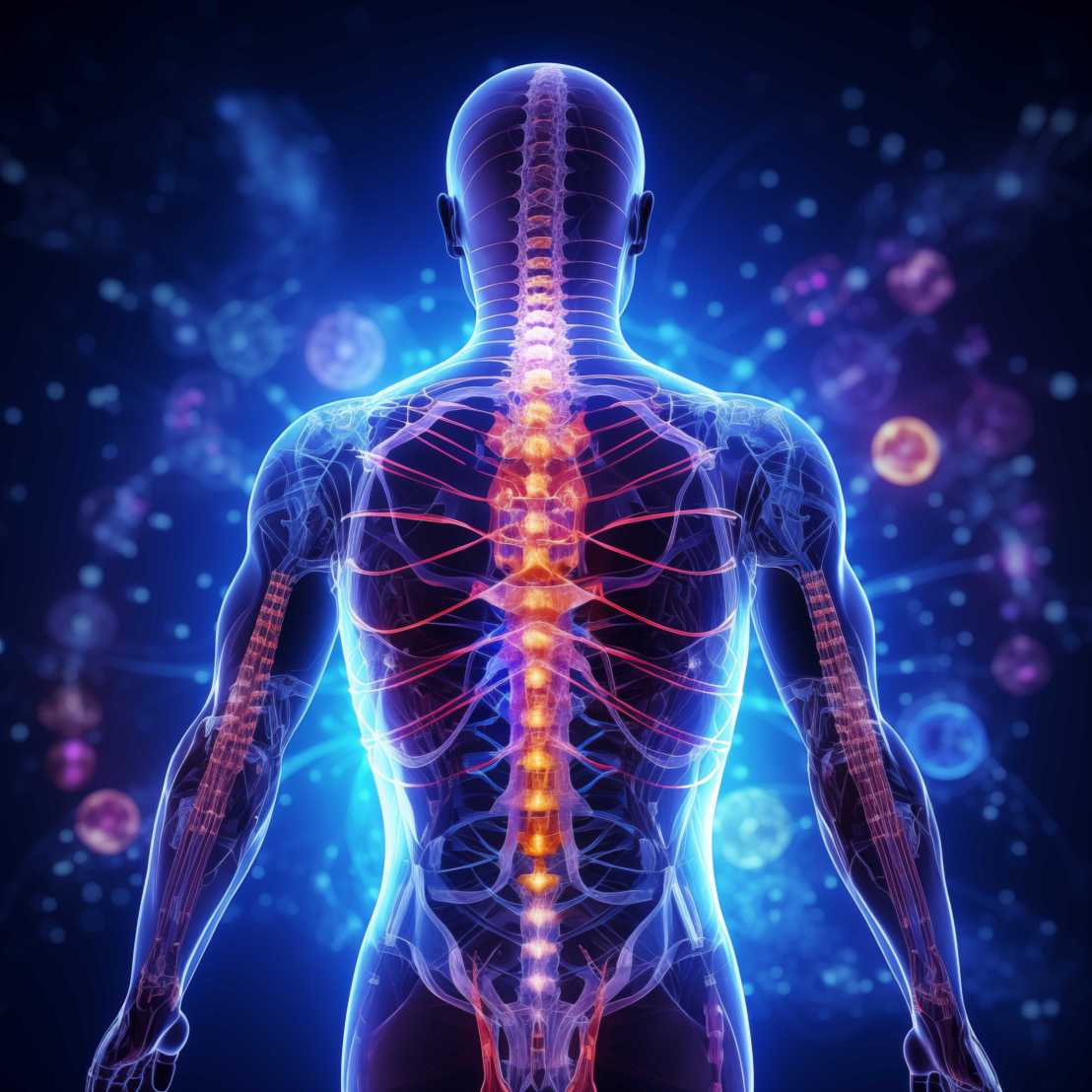
Student
Adrian Kaufmann
external page LinkedIn Adrian Kaufmann
Supervisors
Louis Lukas
Summary
In this thesis, Adrian is working on graph-based subphenotypoing and low dimensional representation of patient trajectories. Additionally, his project aims to explore novel metrics for assessing and predicting recovery after spinal cord injury.
Joint Representation Learning for Oncology Application
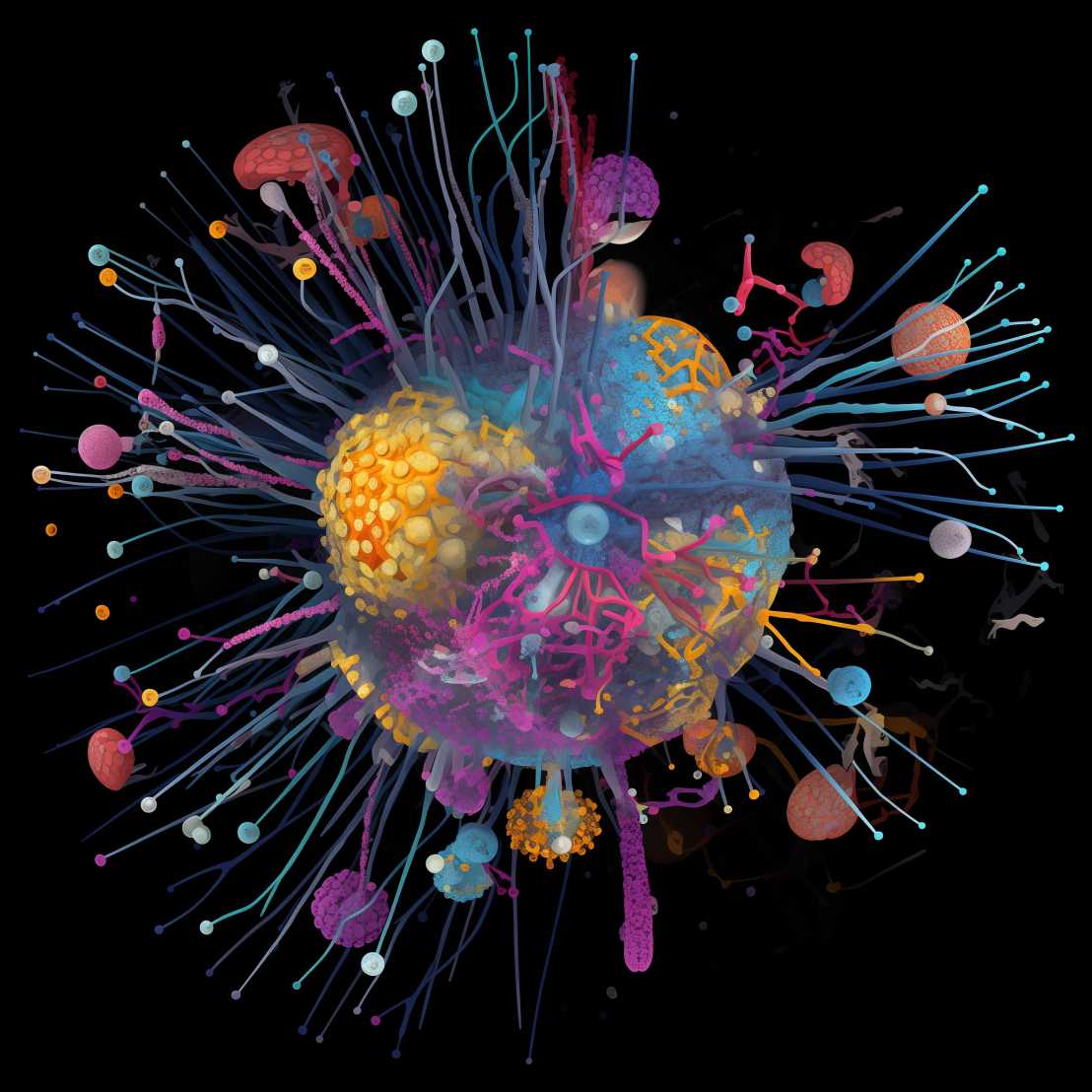
Student
Tanya Nandan
external page LinkedIn Tanya Nandan
Supervisors
Sarah Brueningk, Samuel Håkansson
Summary
In this thesis, Tanya employs a multi-modal approach, integrating various data modalities such as MRI imaging, RNAseq, and Copy Number Variation (CNV) to offer a more comprehensive understanding of brain tumors. Two recently published unsupervised machine learning algorithms, namely Multi-view Spectral Clustering and Joint Multidimensional Scaling, are being implemented. The objective is to derive meaningful joint representations of image and sequencing data. The overarching goal is to identify specific subcategories of tumors and unveil corresponding biomarkers that play pivotal roles in prognosis and therapy sensitivity.
Automatic Tissue Diffusion Segmentation from Multimodal Lumbar Spine Magnetic Resonance Imaging (MRI)
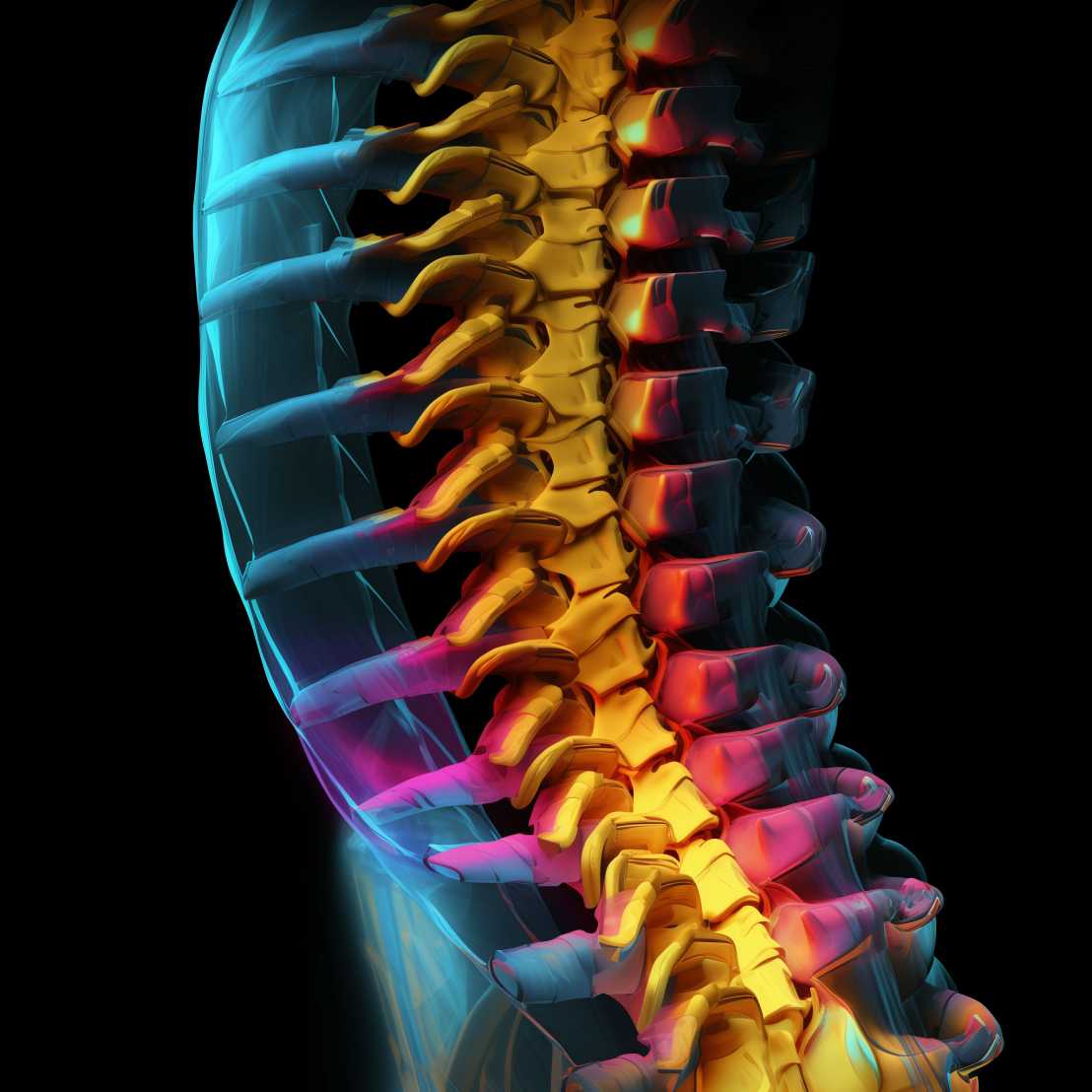
Student
Thomas Iff
external page LinkedIn Thomas Iff
Supervisor
Maria Monzon
Summary
The thesis of Thomas focuses on automating the multiclas image segmentation of multimodal magnetic resonance images of the spine. Our goal is to develop an algorithm grounded in diffusion models and subsequently assess its performance by comparing it to the current state-of-the-art methods employed for this specific task.
Image-based Predictions of Radiotherapy Response in Paediatric Diffuse Midline Glioma
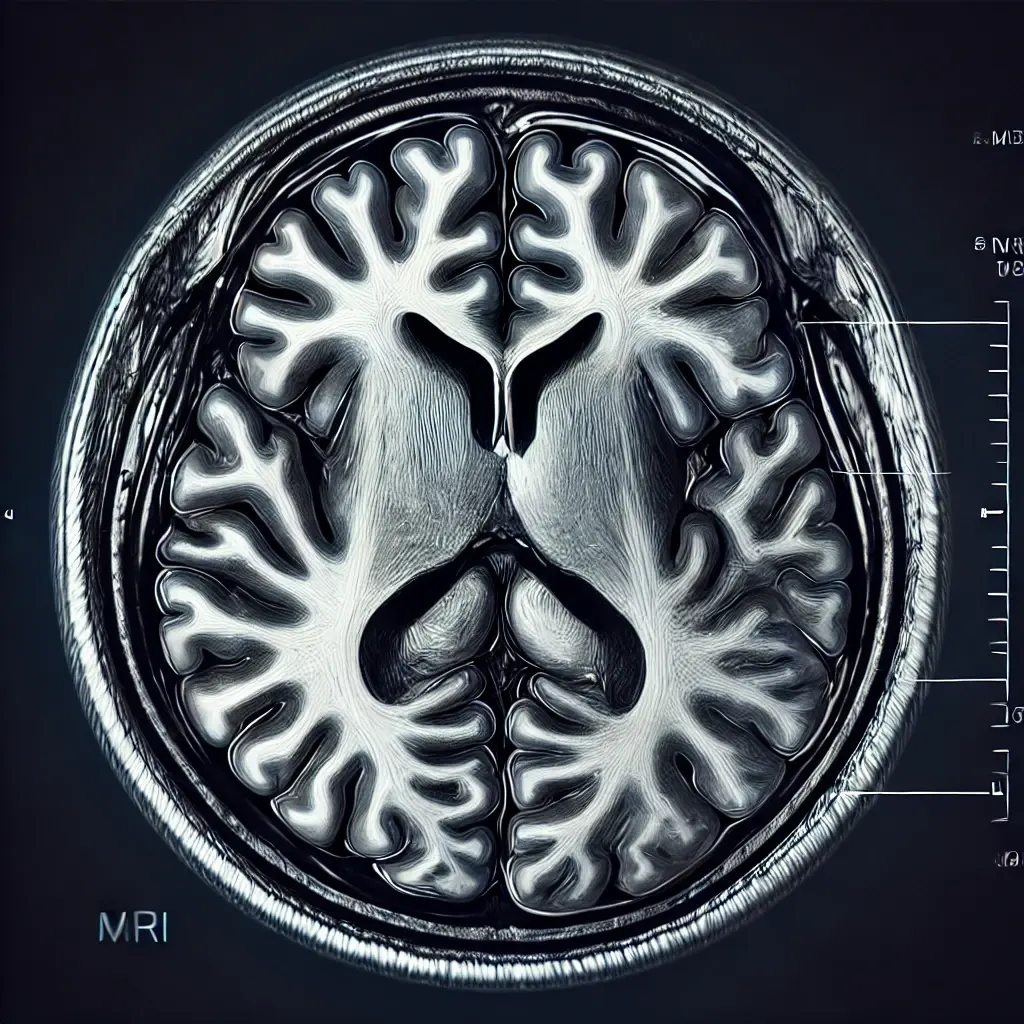
Student
Julian Ortner
external page LinkedIn Julian Ortner
Supervisor
Sarah Brüningk, Daria Laslo
Summary
The master's thesis of Julian revolves around predicting radiotherapy response in pediatric diffuse midline glioma from non-invasive magnetic resonance imaging acquired before radiotherapy. To this end, different data-driven evaluation methods, both supervised and unsupervised, will be explored.
Prediction of Lumbar Spinal Stenosis from MRI-Images with Focus on Explainability for Clinical Application
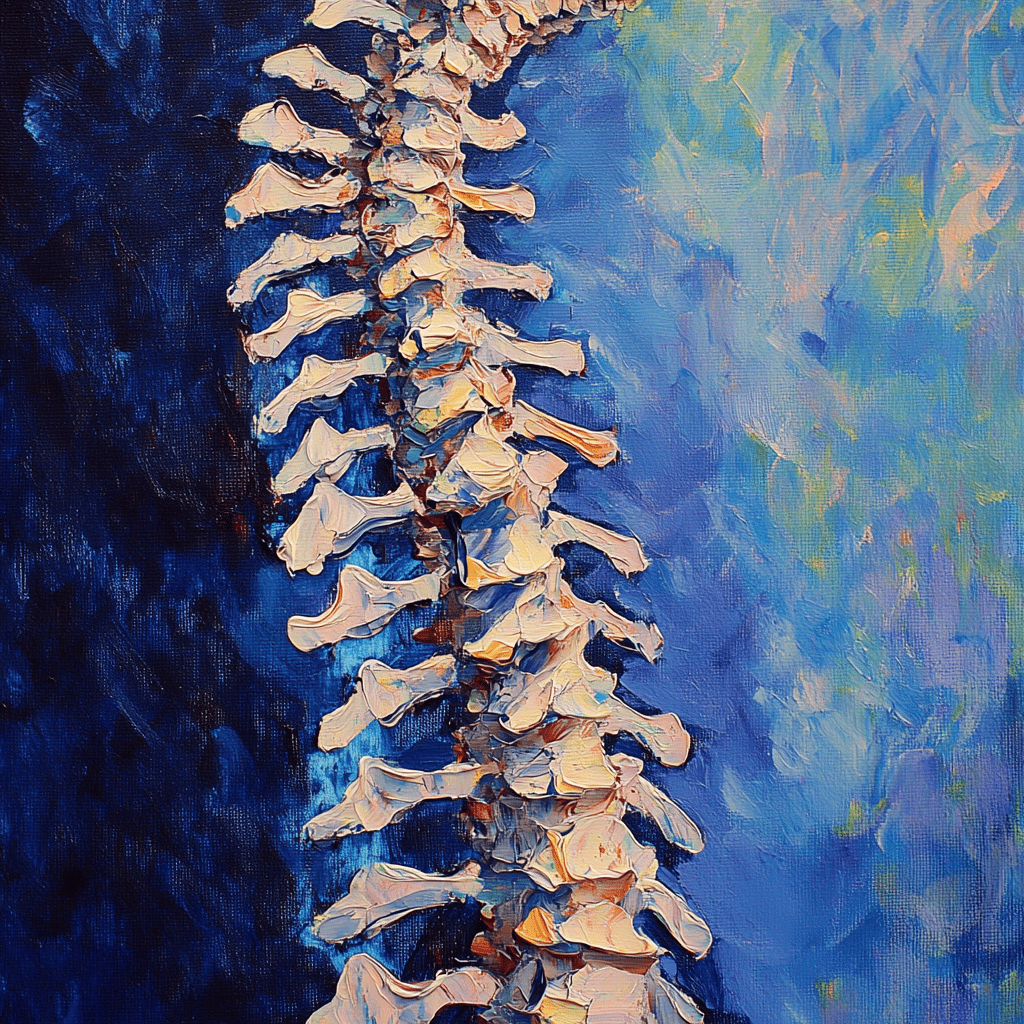
Student
Adriana Brenzikofer
external page LinkedIn Adriana Brenzikofer
Supervisor
Andrea Cina, Maria Monzon
Summary
In her masters thesis, Adriana focuses on the prediction of Lumbar Spinal Stenosis (LSS) from MRI Images using Convolutional Neural Networks (CNN’s). Lumbar spinal stenosis, a common degenerative condition affecting the spine, can lead to significant pain and disability, making early and accurate diagnosis critical. Additionally, this work will address the challenge of applying deep learning models to real-world clinical settings with a focus on explainability and uncertainty estimation. This approach will allow clinicians to gain insights into model’s decision-making process and assess the reliability of its predictions.