Maria Monzon Ronda
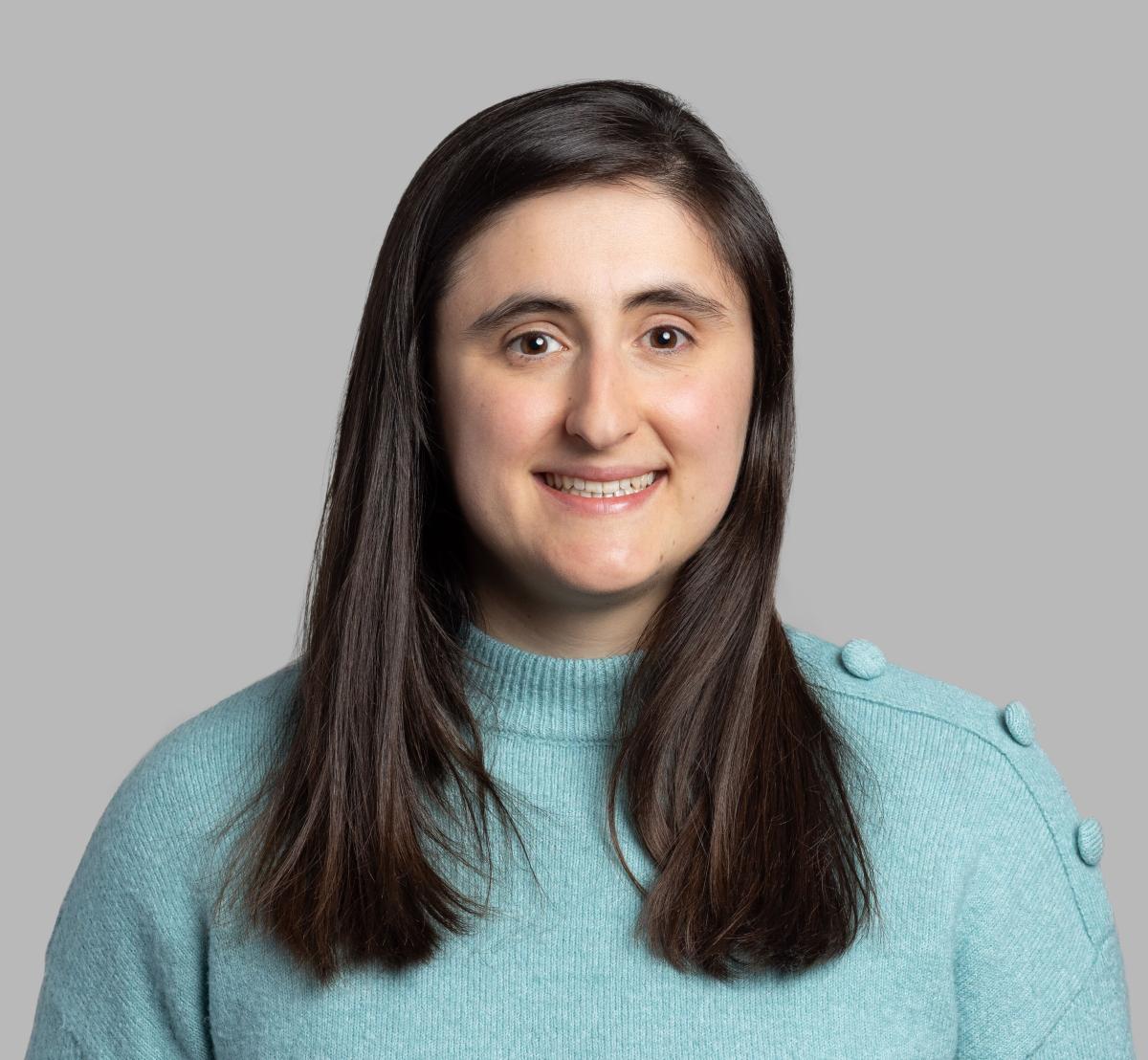
Maria Monzon Ronda
Student / Programme Doctorate at D-HEST
Additional information
Research area
Low back pain (LBP) is a common cause of disability [1] that affects a significant portion of the world's population, with a prevalence of 8.5%. Although steroid injection therapy is often used in clinical practice, its effectiveness largely depends on the underlying cause of LBP [2]. Therefore, the success of injection therapy could be significantly improved if patients with a high probability of response could be selected.
The research question is to evaluate the feasibility of predicting the infiltration outcome to determine whether a patient with LBP will benefit from it.
The objective is to develop a predictive model that accurately predicts the probability of success of injection therapy treatment based on objective and patient-specific characteristics.
We would develop a predictive model by combining multiple sources of health-related data (social, clinical, imaging, gait parameters) using machine learning techniques. Data-driven biomarker discovery would potentially enable distinguishing treatment responders, thus aiding clinical decision-making. The purpose is to improve patient care by leveraging predictive analytics to identify and mitigate infiltration risks at the individual patient level.
The conclusions of the project will make a significant contribution to improving the clinical management of LBP and reducing its economic costs. It could also lead to a re-evaluation of the routine use of MRI.
The personalized predictive model would improve the precision of the evaluation and optimize the selection of treatment for patients with LBP.
Ultimately, personalized assessment can help identify the underlying causes of LBP, informing future research directions and clinical practice.
- J. Hartvigsen, M. J. Hancock, A. Kongsted, Q. Louw, M. L. Ferreira, S. Genevay, D. Hoy, J. Karppinen, G. Pransky, J. Sieper, R. J. Smeets, and M. Underwood, “What low back pain is and why we need to pay attention,” Lancet, vol. 391, pp. 2356–2367, 6 2018.
- B. W. Koes, R. J. Scholten, J. M. Mens, and L. M. Bouter, “Efficacy of epidural steroid injections for low-back pain and sciatica: a systematic review of randomized clinical trials,” Pain, vol. 63, pp. 279–288, 12 1995
Maria Monzon is PhD candidate at ETH working in the intersection of data science, neuroimaging and neurology. Previously, she worked as Computer Vision Researcher on Advance Image Analysis Group at BASF. Her aim is to develop robust and trustworthy Deep Learning algorithms for solving computer vision problems in Bioscience.
She was granted a study scholarship for graduating with honors in highschool. She received his B.Sc. in Telecommunication Technology Engineering from the Basque Country University (UPV-EHU), including one semester abroad in Germany with the help of an Erasmus scholarship. She completed her bachelor thesis at BioRes group with excellent grade. In parallel, she attended a micro-master in Formation and Entrepreneurship in Biomedical Engineering (UPV-EHU).
Right after, she moved to Germany to pursue an M.Sc. in Biomedical Engineering at Friedrich-Alexander University Erlangen-Nürnberg (FAU) in data and image processing major. During her studies, Maria worked on multiple university-related and personal research projects in the areas of Deep Learning for Computer Vision and Biomedical Image and Signal analysis. She completed her master thesis at Siemens Healthineers, where she worked with PD. Dr. Daniel Giese and Prof. Andreas Maier on developing novel deep learning algorithms assessment of cardiac valve motion on time-resolved Magnetic Resonance Images. After her master, Maria did an Erasmus+ internship at Bitbrain Technologies, where she developed machine learning algorithms for decoding grasping action from EEG brain signals. Since then, Maria has worked as a research engineer at BASF R&D, where she developed state-of-the-art deep learning algorithms to solve Computational Life Science Problems. For more informatin on her research experience see Experience & Projects
Her current research interests include Computer Vision, Biomedical Image Analysis, Trustworhty Deep Learning and Machine Learning for Healthcare. She cares about efficient coding and reproducible research.
Membership
Since | Membership |
---|---|
2020 | IEEE |
Additional information
For further details refer to Personal Webpage