BMC Article on Studying Missingness in SCI Data: Challenges and Impact of Data Imputation
In the age of big data, while advanced mathematical and statistical models show promises in understanding patient recovery, fields studying rare conditions like spinal cord injury (SCI) and rehabilitation face challenges due to small sample sizes and missing data, hindering comprehensive analysis.
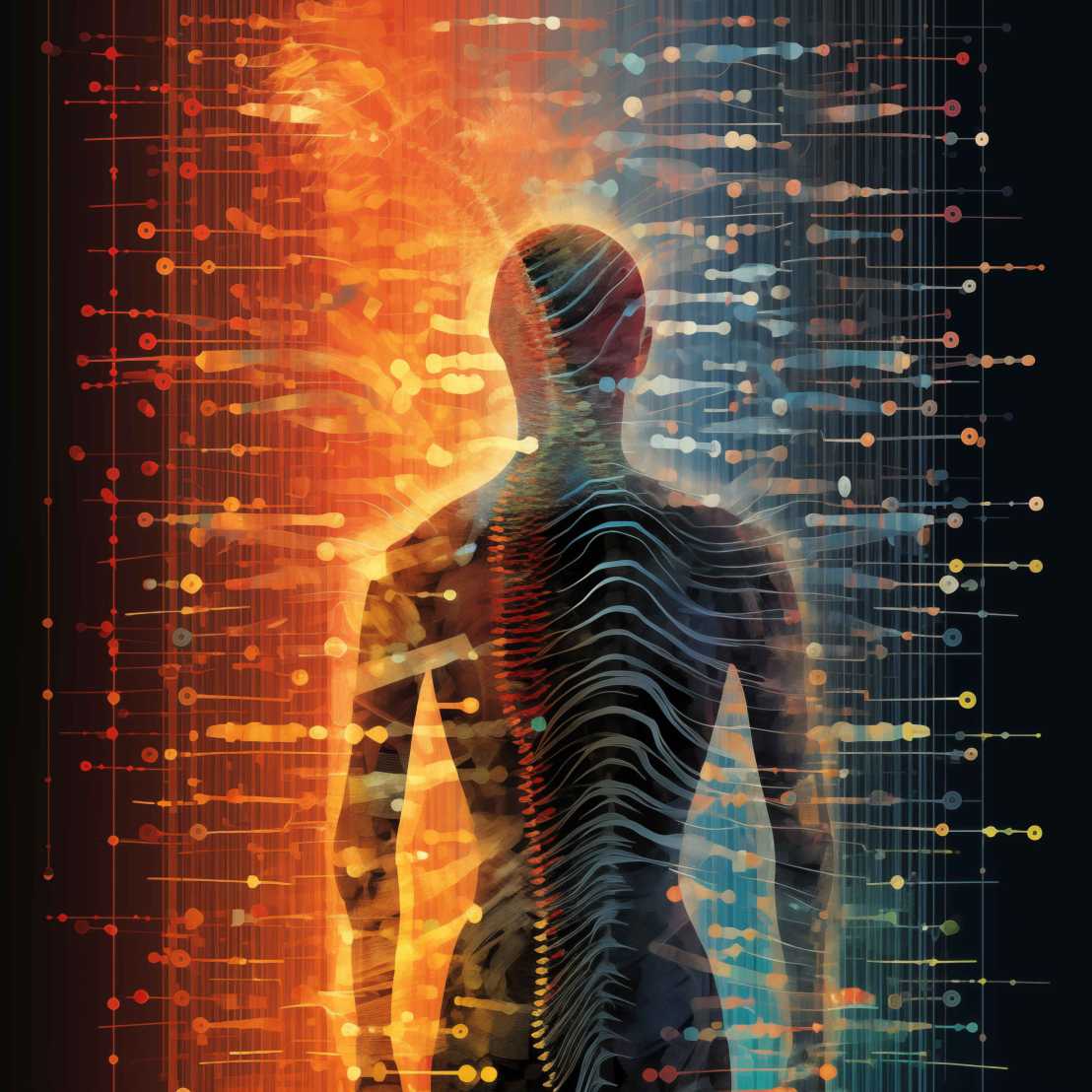
There exist multiple ways of handling missing data. E.g., removing patients with missing data or imputation. Imputation refers to the procedure of inferring likely values of the missing entries.
In traumatic SCI disease progression, patients will recover to some extent over time. Most of the recovery takes place in the first 6 months after injury followed by a plateau between 6 and 12 months after injury. The recovery is characterized by non-linear and highly heterogeneous recovery patterns. Owing to a scarcity of studies, the effect of missing data and imputation is not well understood for SCI datasets.
Our lab members Lucie Bourguignon, Louis Philipp Lukas, Sarah Brüningk, Catherine Jutzeler and colleagues delved into this matter.
In their simulations, they discovered that using mean imputation can significantly skew results away from the expected outcomes. When dealing with missing data in later stages (6 months or more after injury in this study), last observation carried forward (LOCF) appears to be the better choice for imputation. The study highlighted that a universal imputation approach is insufficient for SCI datasets.
Tailored strategies for missing data, like characterising the missingness pattern and using LOCF for repeated measures evolving to a plateau over time, are essential. Clear and consistent reporting of missing data details is crucial for improving research interpretation, transparency, and reproducibility. These results can be applied beyond SCI to other medical scenarios like stroke , traumatic brain injury and MS rehabilitation which show similar plateau, scarcity of datasets and missing data.
The scientific article of external page Bourguignon L., et al. 2024.
Visit also our external page LinkedIn BMDS for more news.