Paediatric Sepsis
Detection and Progression
Sepsis is a life-threatening medical condition, characterised by a dysregulated response to infection that leads to organ dysfunction. It is a significant cause of death and disability in children and adults, and remains a leading cause of death worldwide. Current diagnostic methods and recognition criteria for sepsis are limited by their low sensitivity and slow results, leading to delays in accurate diagnosis and treatment, especially in children who may initially show symptoms resembling mild viral illnesses. Therefore, there is a critical need for faster and more reliable sepsis diagnoses to improve patient outcomes, reduce costs, and prevent inappropriate use of antibiotics, especially in the era of emerging drug-resistant pathogens.
Despite its global prevalence, the biological mechanisms of sepsis are poorly understood, and as a result, currently there are no dependable biomarkers to accurately identify the dysregulated host response which characterise sepsis, particularly in children. Currently, clinicians measure disease severity by the different levels of organ dysfunction using established scoring systems, such as the Sequential Organ Failure Assessment (SOFA) and Paediatric Logistic Organ Dysfunction (PELOD and PELOD-2) scores, which are inherently unreliable and do not lend themselves to an early detection of sepsis.
In recent years, advances in omics technologies, including transcriptomics, proteomics and metabolomics, have offered the potential to systematically study and identify distinct host response patterns in sepsis. Moreover, machine learning (ML) applications in both medicine and sepsis specifically have led to remarkable advancements that can accelerate research and improve clinical practice.
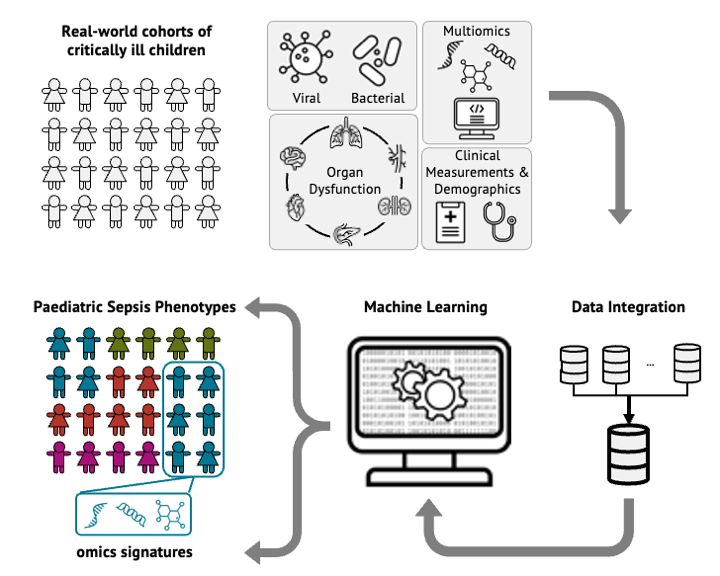
Identification of paediatric sepsis phenotypes
By integrating demographics, clinical information and multi-omics data from real world paediatric cohorts and using machine learning methods, we aim to identify sepsis phenotypes and sub-phenotypes that can shed light into the biological mechanisms underlying sepsis progression. This can further improve our understanding of the progression from an infection without any complications to sepsis and multi-organ dysfunction.
Discovery of paediatric sepsis biomarkers
By analysing the complex molecular signatures, associated with sepsis across the different phenotypes, we aim to uncover hidden patterns and prioritise key molecular features indicative of the disease severity. The use of machine learning algorithms that can capture and highlight these patterns, can potentially provide a comprehensive understanding of paediatric sepsis and lead to an earlier diagnosis and a personalised treatment.