Paediatric Oncology
Prognosis and Personalised Treatment
Amongst different tumor entities, pediatric diffuse midline glioma (DMG) stands out with a particularly poor prognosis, exacerbated by patients’ ineligibility for surgery. Radiotherapy remains the standard of palliative care for DMGs, making it essential to maximize its benefits for the individual. Our group investigates different approaches to personalize and maximize treatment benefits for pediatric brain tumor patients with a focus on pediatric DMG. The data required for these projects is provided as part of an international network of collaborators.
Predicting therapy response from multi-omics inputs
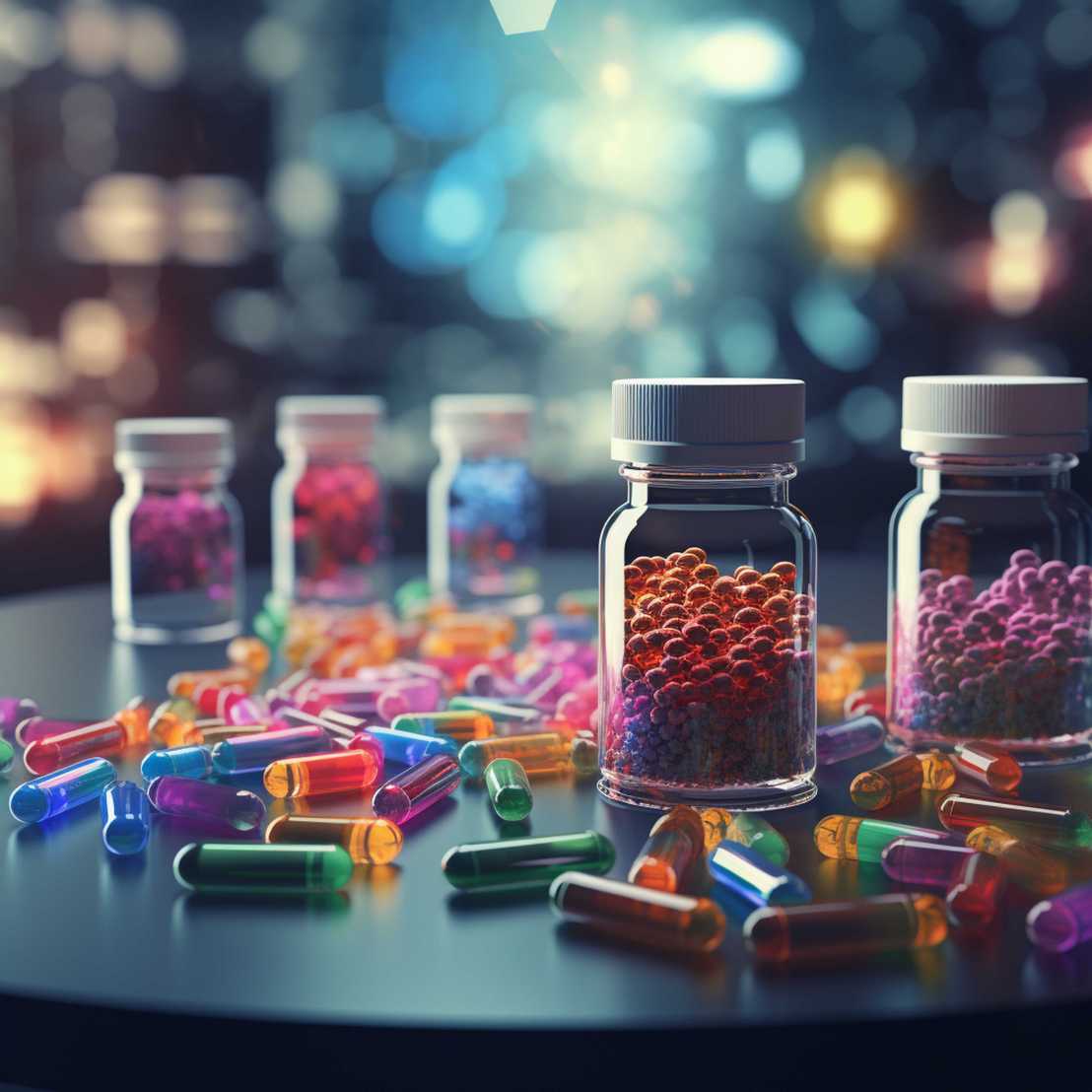
Cancer's complexity challenges treatment, exacerbated by mutations and environmental factors. Pediatric cancer care, less common but unique, faces hurdles including long-term side effects and limited targeted therapies.
Machine learning advances personalized medicine, using genomics and other data to predict drug responses. This study evaluates models like DROEG and PaccMann, identifying effective techniques for data extraction. Two models are developed: 'One Drug at a Time' for individual predictions and a holistic model for simultaneous training on diverse drug responses.
Applied to pediatric cancer cell lines, these models improve prediction accuracy. The 'One Drug at a Time' model excels in new cell line predictions, while the holistic model outperforms in new drug response predictions. This evaluation underscores the importance of varied training inputs and methodologies, offering insights for future advancements.
Personalized radiotherapy response prediction from imaging
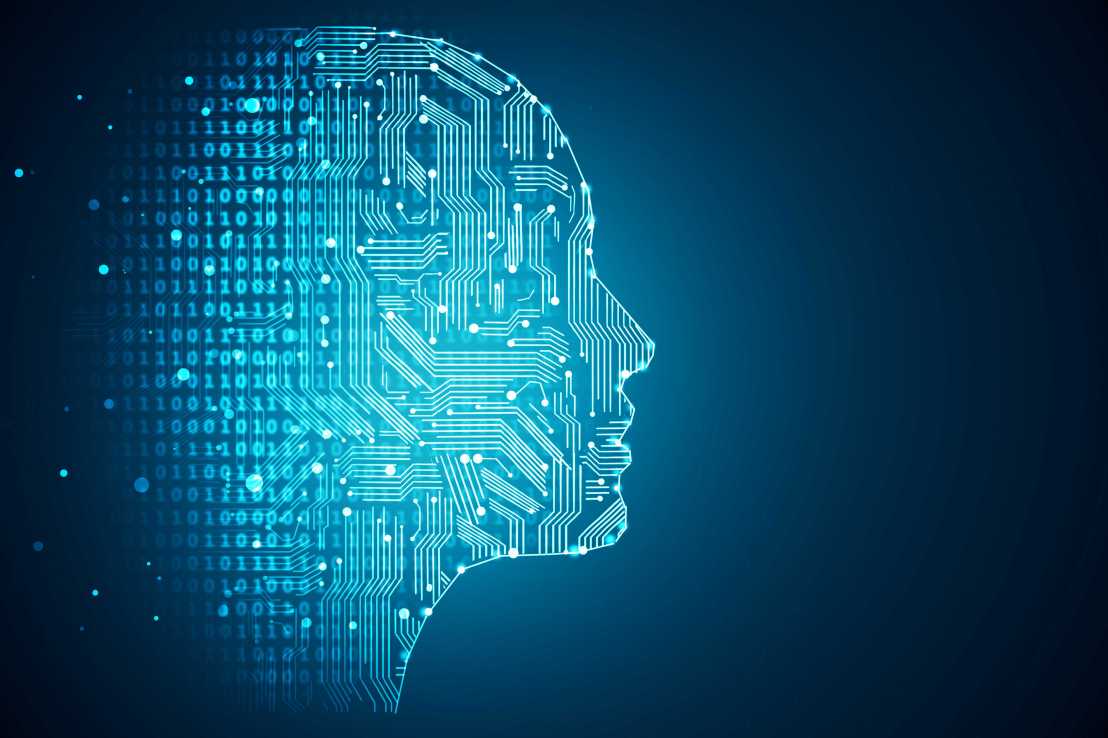
Diffuse midline glioma (DMG), a primary tumor within the most sensitive part of the brain, is a fatal disease primarily affecting children between 4-7 years of age. Owing to the delicate location and aggressiveness of these tumors, treatment options and surgical interventions are greatly limited and DMG patients die within two year of diagnosis.
One key life-prolonging and palliative treatment is radiotherapy (RT). However, not every child responds equally to the current one-size-fits all therapy, which is mostly based on clinical experience in adults. The efficacy of RT may hence be improved by tailoring it to the clinical needs of individual pediatric patients. Since tumor anatomical location hinders an invasive biopsy, we propose to investigate whether non-invasive magnetic resonance imaging could be used to infer the tumor's radiosensitivity.
Here, we propose a combinatorial approach by employing two powerful tools originating from different research fields: machine learning and modelling of tumor growth with differential equations. This combination of models is unique to the expertise of our team and facilitates not only to identify which child will benefit from conventional RT, but also if and how the impact of RT can be maximized by changing its scheduling and dosing. By evaluating the criteria based on which the algorithm made the decision for effective or non-effective RT we can infer information on the underlying tumor characteristics and how these are represented in the images.
This will improve our biological understanding of DMGs and could provide indications of which other therapies to combine with RT in order to improve the tumor's radiosensitivity. As such, we provide a digital health tool to guide doctors in their choice of the optimal treatment strategy to improve the quality of life for children and their families faced with the devastating diagnosis of DMG. Since our approach investigates cost-effective and broadly available RT and does not require biopsy information, it is readily translatable to clinics worldwide without the need for a specialized treatment center.
Predicting Anatomical Tumor Growth in Pediatric High-grade Gliomas using Denoising Diffusion Models
Apart from defining the gross tumor volume, identifying high-risk brain regions of likely disease progression could guide the design of personally adapted geometric radiotherapy dose distributions. Currently, the pattern of anatomical tumor progression estimation is driven by the clinician’s experience in the context of an individual patient. Data-driven models informing on the location and expected rate of progression could hence greatly improve these personalized predictions and guide localized therapies.
In this project, we use generative computer vision models to predict clinically relevant anatomical representations of brain tumor progression on magnetic resonance images (MRIs).
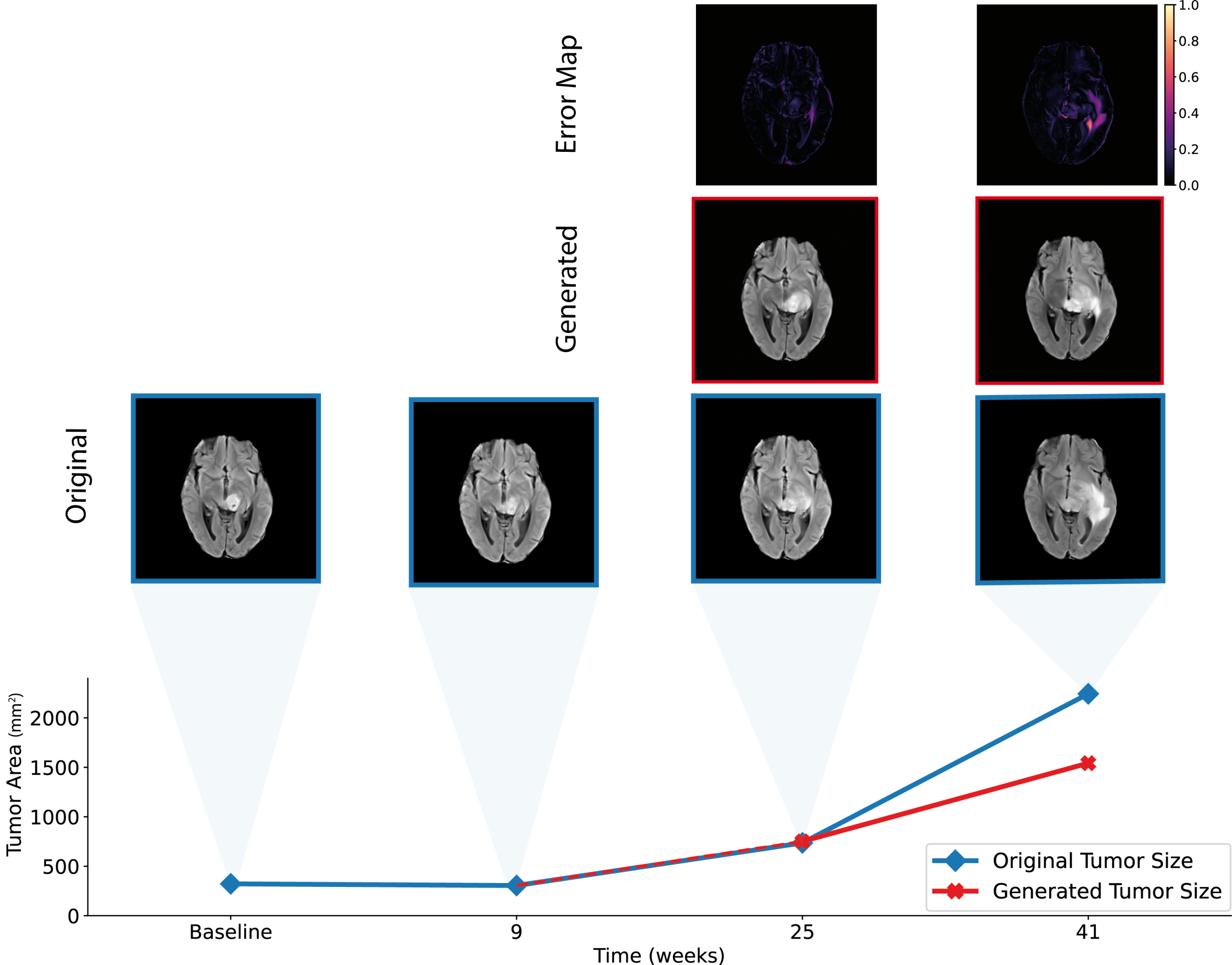
University of California San Francisco, Kinderspital Zurich, DMG Center Zurich, Yale School of Medicine, University of Pennsylvania, Pacific NeuroOncology Consortium.