Lower Back Pain
Prediction and Progression
Low back pain (LBP) is the leading cause of disability worldwide, affecting over 600 million of the global population at some point in their lifetime (i.e. 80–85% of people of all age groups can experience LBP at some point in their lives). It is predicted that this number will rise to 843 million people by 2050, resulting in a substantial economic burden on individuals and society. Beyond their immediate health consequences, the societal and economic costs are of epidemic proportion and thus, make LBP a global public health priority. Despite its highly heterogeneous nature, LBP continues to be treated as a single disease entity. Treatment selection is based upon trial and error, rendering the therapeutic process highly dissatisfying for patients and doctors alike.
Predicting individual treatment response for injection therapy
Steroid injection therapy is often used in clinical practice to treat LBP, but its effectiveness largely depends on the underlying cause of LBP. Therefore, the success of injection therapy could be significantly improved if patients with a high probability of response could be selected.
The objective of the project is to develop a machine learning model that accurately predicts the probability of success of injection therapy treatment based on objective and patient-specific characteristics.
The personalized predictive model would improve the low back pain assessment and optimize the selection of treatment for patients with LBP. The conclusions of the project can help identify the underlying causes of LBP, informing future research directions and clinical practice.
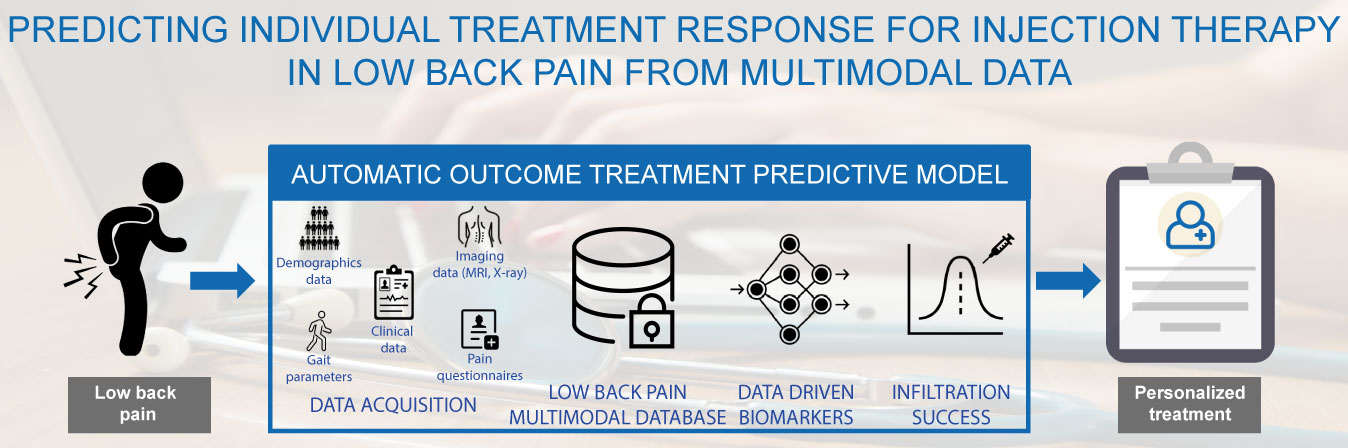
Minimal Clinically Important Change
The Minimal Clinically Important Change (MCIC) concept has gained significant attention in the field of Patient-Reported Outcome Measures (PROMs). The MCIC is often used in conjunction with PROMs to interpret the scores from these measures and the clinical relevance of changes in health status, for example the improvement after treatment. The MCIC provides a benchmark to determine whether a change in score, is not just statistically significant but also clinically meaningful from the patient’s perspective. The relevance of MCIC has been recognized particularly in the context of the Core Outcome Measures Index (COMI), a multidimensional questionnaire used to assess patient-reported outcomes in spinal disorders. MCIC helps clinicians and researchers interpret changes in COMI scores and understand whether these changes are meaningful to patients. The MCIC used the most in the clinical practice is equal to a 2.2-point decrease in COMI score from baseline to 12 months. This study aims to demonstrate that while the MCIC offers valuable insights into patient-reported outcomes, its interpretation should be approached with caution due to potential variations arising from different calculation methods. Some recommendations on which MCIC values have to be used are given as a general guideline.
Localization of landmarks in the cervical spine
The complexity of the cervical spine alignment originates from the interrelationship between the head, the thoracolumbar alignment as a flexible base, and the lower extremities. The investigation of cervical spine alignment is becoming increasingly valuable as the relationship between proper sagittal alignment and better clinical outcomes is better understood. Multiple cervical spine studies have looked at relationships between cervical alignment and the occurrence of adjacent segmental disease namely complications of spinal fusion, and studies over the last several years have revealed the relationship with health-related quality of life (HRQOL). The purpose of this study was to develop a new automated image analysis algorithm for the comprehensive measurement of cervical spine alignment parameters in lateral radiographs and to evaluate its accuracy in comparison to human examiners on an external validation set. In particular, starting from the model, we developed and validated a deep learning model to automatically calculate cervical spine landmarks, with the overarching goal to compute a comprehensive set of cervical spine alignment parameters from these landmarks.
- external page call_made Dr. Fabio Galbusera, Head of the Spine Group, Schulthess Klinik
- external page call_made Dr. Jacopo Vitale, Research Associate Spine Group, Schulthess Klinik
- external page call_made Dr. med. Zina-Mary Manjaly, Head of the Human Performance Lab, Schulthess Klinik
- chevron_right Prof. Dr. Ender Konukoglu, Biomedical Image Computing, ETH Zurich
- external page call_made Dave O’Riordan, Senior Data Manager Spine Group, Schulthess Klinik
- external page call_made Réka Enz, Research Assistant Neurology Department, Schulthess Klinik